Machine learning tools for video interviewing platforms have been on the receiving end of sharp criticism, and not without justification. Some technology within the budding sector has demonstrated biases, making for problematic hiring practices, but tech is only as empathic as the people who build and maintain it, and our call-to-action at myInterview has always been to build new kinds of systems from the ground up to help foster diversity and uphold candidate experience.
The Questions & The Observations
To address the doubts and questions that have arisen around automated candidate review, particularly in regards to personality assessment, the need became clear for a thorough scientific study on the effectiveness and fairness of these high-potential solutions. The remote conditions of the pandemic highlighted the immense benefits of video interview solutions. It took their use to new heights, but along with the heightened potential of these tools, so too is there a heightened imperative for greater transparency into how these systems fare when compared to traditional methods of reviews conducted by trained personnel.
With this study, we set out to determine the ability of machine learning algorithms to replicate the Observe, Record, Classify and Evaluate (ORCE) system. ORCE is an assessment approach, traditionally carried out by multiple expert raters, that evaluates interview behavior against specific, predetermined job dimensions and competencies.
This methodology can measure how well candidates perform throughout an interview, gather relevant personality data and ultimately generate fair assessments for all applicants. By focusing on observable insights that are transparent and clear for all decision-makers, the steps of this methodology can mitigate the possibility of discrepancies, disregarded information or misremembered data.
A key piece of our study was the involvement of two expert raters with extensive ORCE training and experience in psychology, personality assessment and data evaluation. We asked these experts to manually review 4,512 recorded interviews and rank them according to the Big 5 Factors of Personality (Openness, Conscientiousness, Extroversion, Agreeableness, Neuroticism) based on evidence of behaviors presented through both interview content and candidate intonation.
This step of the process provided a dataset against which we could then compare the machine learning solutions. Those same 4,512 interviews, selected randomly from a range of job openings with a diverse set of applicants from the UK, Australia, USA and Mexico, were subsequently analyzed by myInterview’s Machine Learning-powered ranking algorithm.
Analyzing the Data
Our algorithm yielded an average correlation of 0.452 across the Big 5 dimensions. This represents a healthy result, albeit with room to improve, for a first-of-its-kind study. The results are promising, as frankly, the correlation rates were higher than we had expected, demonstrating that the overall accuracy of the personality assessment can already be trusted at the current levels of development. Having this benchmark and data provides context for the current state of automated hiring and sets a high bar for operational expectations across the industry.
One of our primary takeaways from this study was that these tools can not only assess personality in job candidates effectively and efficiently, but they have the ability to reduce the potential for biased input on the part of both candidates and hiring managers, as well.
That’s good news for the HR industry but even better news for job candidates hoping to be evaluated on their own merits. We firmly believe that automated personality assessments have the potential to eliminate two of the major issues that plague traditional personality assessment methods.
First, self-reporting questionnaires depend on any given candidate to have a high enough level of self-awareness and personal insight to accurately report on their behaviors. Second, reviewer biases during the interview process are difficult to mitigate. Growing public concern about machine learning perpetuating the same mistakes as human assessors is valid, but it overlooks the fact that a standardized automated process with long-term monitoring allows for methodical, data-informed system updates.
This is to say that while automated hiring tech may be born with the same level of fallibility as its human counterparts, it is also born with the possibility of even greater accuracy through the process of improvement over time
Conclusions & Confidence
Once again, tech is only as empathic as the people who build and operate it. To build a hiring platform that promotes diversity, one must keep diversity in mind while building and using every element throughout the solution, from the tagging teams who sort the data, to the developers who design the system, to the machine learning experts who monitor for problems at every step of the process.
As the hiring market swells alongside economic recovery, the rise of streamlined automated processes that can be conducted in the fairest way possible should be extremely encouraging to both job seekers and organizations looking to grow. We hope that the scientific backing of this method, as determined by the experts in our study, will allow organizations to optimize hiring and bolster company culture by adopting the latest in AI technologies while remaining confident that they are making the best, fairest hiring decisions possible.
Authors
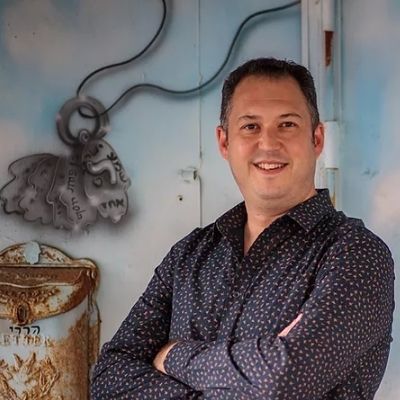
Clayton Donnelly
Experienced Human Capital consultant with a demonstrated history of working in the FMCG, Retail, Mining and Financial Services industries. Skilled in Leadership Development, Culture & Team Development, OD Consulting, Coaching, and Psychological Assessment. A practical psychologist that is passionate about unlocking potential.
Recruit Smarter
Weekly news and industry insights delivered straight to your inbox.